
Author: Paul Gustafson
Edition: 1
Binding: Hardcover
ISBN: 1584883359
Mismeasurement of explanatory variables is a common hazard when using statistical modeling techniques, and particularly so in fields such as biostatistics and epidemiology where perceived risk factors cannot always be measured accurately. Download Measurement Error and Misclassification in Statistics and Epidemiology: Impacts and Bayesian Adjustments from rapidshare, mediafire, 4shared. With this perspective and a focus on both continuous and categorical variables, Measurement Error and Misclassification in Statistics and Epidemiology: Impacts and Bayesian Adjustments examines the consequences and Bayesian remedies in those cases where the explanatory variable cannot be measured with precision.
The author explores both measurement error in continuous variables and misclassification in discrete variables, and shows how Bayesian methods might be used to allow for mismeasurement. A broad Search and find a lot of medical books in many category availabe for free download. Measurement Error and Misclassification in Statistics and Epidemiology medical books pdf for free. With this perspective and a focus on both continuous and categorical variables, Measurement Error and Misclassification in Statistics and Epidemiology: Impacts and Bayesian Adjustments examines the consequences and Bayesian remedies in those cases where the explanatory variable cannot be measured with precision A broad
Edition: 1
Binding: Hardcover
ISBN: 1584883359
Mismeasurement of explanatory variables is a common hazard when using statistical modeling techniques, and particularly so in fields such as biostatistics and epidemiology where perceived risk factors cannot always be measured accurately. Download Measurement Error and Misclassification in Statistics and Epidemiology: Impacts and Bayesian Adjustments from rapidshare, mediafire, 4shared. With this perspective and a focus on both continuous and categorical variables, Measurement Error and Misclassification in Statistics and Epidemiology: Impacts and Bayesian Adjustments examines the consequences and Bayesian remedies in those cases where the explanatory variable cannot be measured with precision.
The author explores both measurement error in continuous variables and misclassification in discrete variables, and shows how Bayesian methods might be used to allow for mismeasurement. A broad Search and find a lot of medical books in many category availabe for free download. Measurement Error and Misclassification in Statistics and Epidemiology medical books pdf for free. With this perspective and a focus on both continuous and categorical variables, Measurement Error and Misclassification in Statistics and Epidemiology: Impacts and Bayesian Adjustments examines the consequences and Bayesian remedies in those cases where the explanatory variable cannot be measured with precision A broad
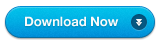
Related books

The Workflow of Data Analysis Using Stata
The Workflow of Data Analysis Using Stata, by J. Scott Long, is an essential productivity tool for data analysts. Long presents lessons gained from his experience and demonstrates how to design and implement efficient workflows for b


Measurement Error: Models, Methods, and Applications (Chapman & Hall/CRC Interdisciplinary Statistics)
Over the last 20 years, comprehensive strategies for treating measurement error in complex models and accounting for the use of extra data to estimate measurement error parameters have emerged. Focusing on both established and novel approaches,


Targeted Learning: Causal Inference for Observational and Experimental Data (Springer Series in Statistics)
Establishes causal inference methodology that incorporates the benefits of machine learning with statistical inferencePresentation combines accessibility with the method's rigorous grounding in statistical theoryDemonstrates targeted learning in epid

Tidak ada komentar:
Posting Komentar